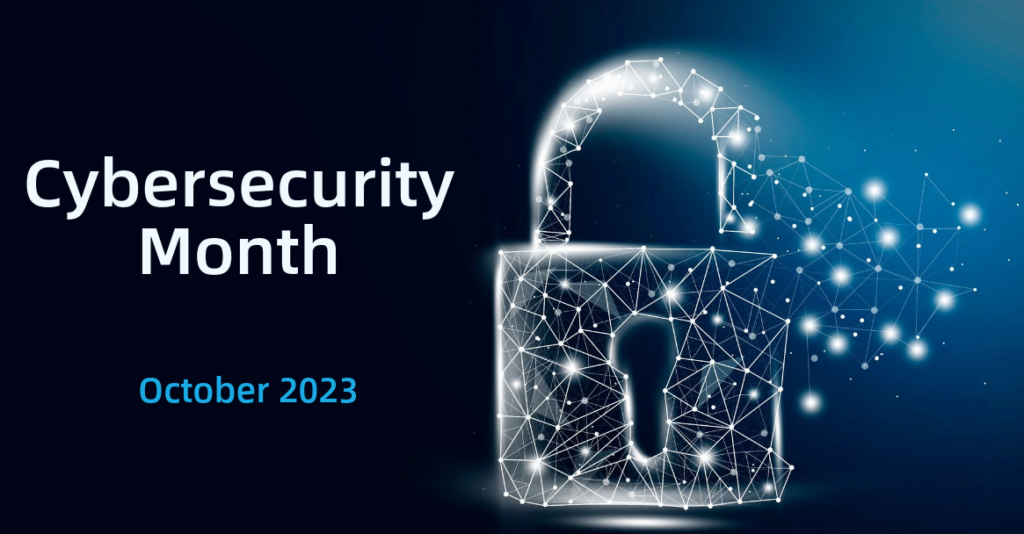
Cyber Threat intelligence
The M4D Cyber Threat Intelligence (CTI) tool enables the collection, storage, analysis, correlation, and sharing of information about threats and vulnerabilities from both internal and external sources; such information is identified and analysed using AI technologies in order to extract knowledge regarding attackers and any other abnormal behaviour.
Internal sources comprise honeypot instances, firewalls, Security Information and Event Management (SIEM), Intrusion Detection Systems (IDSs) and more. External sources include among others vulnerability databases, CERT feeds, databases with Proof-of-Concept (PoC) exploits, social media platforms, as well as various sources from both Surface and Dark Web.
Using rule-based and machine learning-based analysis techniques CTI is extracted. For example, machine learning-based techniques, such as pattern identification and anomaly detection, can extract valuable CTI from the collected data. Finally, CTI is enriched based on correlation techniques before stored and shared through MISP.
Dynamic Taxonomies & Ontologies
The M4D Dynamic Taxonomies and Ontologies tool is the outcome of the integration of two solutions.
The first is responsible for the generation of new taxonomies and the automatic update of existing ones. To achieve this, the BERTopic technique, which has been applied in various fields, such as natural language processing, information retrieval, and data mining to extract meaningful insights from large collections of text data, is employed. It is also responsible for automatically updating existing taxonomies (such as MISP) using sentence-transformer.
The second is responsible for the generation of new ontologies and the merging between ontologies. To achieve this, the REBEL model is used to extract relationships between the different ontologies.
For the entire process to be realised, the M4D CTI tool is used in order, to gather, store, and analyse cyber threat related information.
AI-based Intrusion Detection System
The M4D Collaborative-Intrusion Detection System (C-IDS) integrates well-established signature-based IDS systems with custom-designed anomaly detection Machine Learning-based systems. Furthermore, Federated Learning (FL) is used for creating a collaborative IDS system among organisations. Using this approach, the Machine Learning models can be trained in an effective manner, while sensitive and personal information are kept secure to the data holder.
The aforementioned solutions are being developed, implemented and evaluated in the following EU research projects:
enhances the capabilities of stakeholders, implementing (a) collaborative threat intelligence collection, analysis, and sharing; (b) innovative risk analysis specifically designed for interconnected nodes of an industrial ecosystem; (c) cutting-edge trust and accountability mechanisms for data protection and (d) security awareness training for more informed security choices.
addresses the challenges of IoT- and AI-driven ICT systems through a collaborative-first approach centred around computer security incident response teams (CERTs/CSIRTs). Specifically, the project equips CERTs/CSIRTs with a state-of-the-art incident response toolkit for assessing, detecting, responding to, and sharing information regarding threats and vulnerabilities of IoT and AI-driven ICT systems.
develops a scalable framework for processing data stored in federated cross-border data spaces. The ENCRYPT framework will provide a recommendation engine for end users to personalise their privacy preferences based on the sensitivity of their data and the trade-off between security and performance. In-lab tests and real-world use cases across various sectors (health, cybersecurity and finance) will validate the framework.
combines the two fields of business continuity management (BCM) and cyber threat intelligence (CTI) to generate a situational awareness picture for decision support across all stages of the resilience cycle (prepare, prevent, protect, response, recover).
develops a Water Data Management Ecosystem (WDME) for making data management practices and resources in the water sector accessible, affordable, secure, fair, and easy to use, improving usability of data and the interoperability of data-intensive processes, thus lowering the entry barrier to data spaces, enhancing the resilience of water utilities and boosting the perceived value of data and therefore the market opportunities behind it.
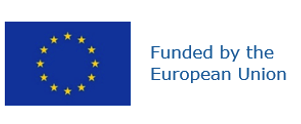
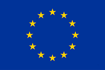