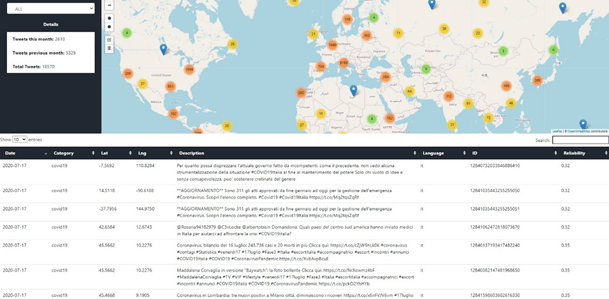
Decision support systems (DSS) have become essential tools for decision-making in critical domains such as healthcare, finance, and defense. These systems provide valuable insights and recommendations that can help responsible personnel make more informed decisions and enhance their situational awareness. However, as Machine Learning models have become more complex, they have become increasingly opaque and difficult for users to understand.
This lack of transparency can lead to a lack of trust in the models and their decisions. To address this issue, it is important for the DSS to be based on explainable AI (XAI) techniques. The ML models should become more transparent and interpretable, allowing the users to understand how a decision was yielded.
This research direction includes machine learning techniques for analyzing and fusing dynamically heterogeneous information obtained from various sources such as Deep Learning and ensemble models. For example, in the disaster management sector, the solutions that we design and develop in the research direction aim
- to classify the severity of a crisis in pre-emergency as well as emergency phase, enhancing the preparedness and response of civil protection authorities, agencies and response services to tackle efficiently a hazardous event
- to facilitate the communication between stakeholders and user interfaces, our activities aim to develop comprehensive and interactive visualisations which will encapsulate high-quality information about hazards, exposure, vulnerability, risks
- to risks maximizing usability, user interfaces will be based on visual analytics creating a strong synergy between the end-user, the analysis methods (e.g., machine learning), and effective visualisations that aggregate and map the risk assessment results in an easy way to non-experts.
- to accurately predict hazardous pathologies for casualties by fusing multiple heterogeneous modalities through state-of-the-art neural network architectures. By integrating data from various sources, such as electronic health records and patient history, the accuracy of early detection and diagnosis of hazardous conditions gets improved.
- to provide explanations about the suggested decisions in order to help the decision makers to make more informed decisions leading to better outcomes. For explaining the black-box models, various methods are used for providing explanations at different levels, namely global and local. Such methods are feature importance techniques, surrogate models, neurosumbolic methods etc.
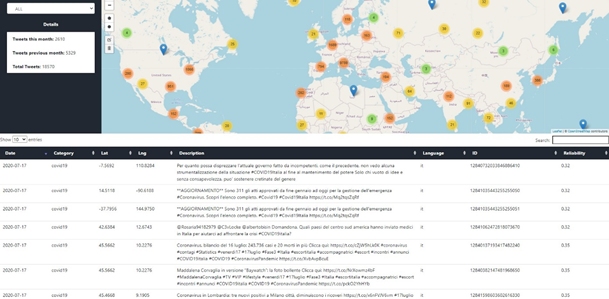
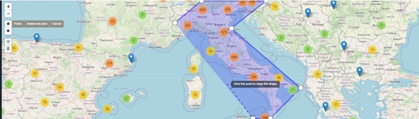